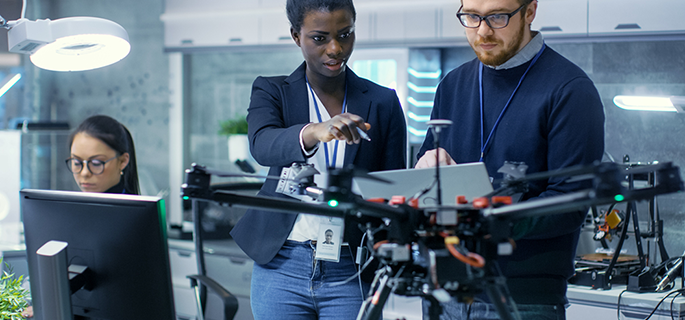
Two years on from the rapid rise of LLM chatbots like ChatGPT, the challenges are starting to show for some customers leveraging generative AI to create new services. Off-the-shelf self-hosted options no longer provide a distinct advantage. Creating custom generative AI models, or even tweaking an existing one, while ensuring low latency, high throughput, and security is often difficult to achieve on existing self-hosted solutions.
But it doesn’t have to be.
Can generative AI deliver for your business?
Senior executives and application developers understand the value that generative AI adds to business but to what extent? Accenture reports that organizations adopting large language models (LLMs) and generative AI are 2.6 times more likely to increase revenue by 10 percent or more.[1]
However, according to Gartner, through 2025, 30 percent of generative AI projects will be abandoned after proof of concept (POC) due to poor data quality, inadequate risk controls, escalating costs, or unclear business value.[2] Perhaps the biggest barrier is the complexity of deploying large-scale generative AI solutions. Fortunately, the market is rapidly adapting and evolving to ease these pain points.
Generative AI is flexible. But it’s not one-size-fits-all.
Most organizations need to deploy a variety of models for generating text, images, video, speech, synthetic data, and more. To successfully launch a generative AI application, developers need to continually test, maintain, and deploy demanding inference workloads at scale. To do this, they’ll choose either to:
- Build, train, and deploy on easy-to-use third-party managed solutions or
- Use a self-hosted solution containing open-source and commercial tools.
Third-party managed generative AI services boast simplicity and user-friendly APIs. However, they can potentially share data externally, which may not meet organizational security policies and procedures.
Alternatively, self-hosted solutions provide better control but are more resource intensive. The downside is that AI models require continuous fine-tuning, custom coding for APIs, and ongoing maintenance and updates.
To achieve the desired outcome for inferencing performance, generative AI models must be optimized to use existing compute capacity and budgets efficiently. This process can be complex and is often time and resource intensive.
Three obstacles to deploying generative AI at scale
- Hitting performance benchmarks Delivering low latency and high token throughput to achieve efficiency and accuracy is essential. Developers need to compress and optimize models using existing infrastructure to deliver a seamless user experience.
- Complexity of deployment Everyone needs a well-supported solution that performs well across different models and applications. Application developers want ease-of-use and consistent APIs while IT teams want standardized, stable, and secure inference platforms.
- Data safety and security Using generative AI responsibly requires a high level of data security. Companies need to protect their data by securing client confidentiality and personally identifiable information (PII) in accordance with in-house security policies and industry regulations.
Efficient generative AI model deployment is critical
To realize the full potential of generative AI, you need to optimize inference. This is where NVIDIA NIM inference microservices is revolutionizing generative AI model deployment. NIM includes a set of production-ready microservices that enable the rapid, reliable, and secure deployment of high-performance generative AI models. NIM gives access to a comprehensive range of industry-standard APIs as well as open-source and custom foundation models from sources like Llama 3, 3.1, and 3.2, Mistral and Mixtral, and Nemotron from NVIDIA.
Performance is a critical benefit of using NIM microservices. For example, the NVIDIA Llama 3.1 8B Instruct NIM has achieved 2.5 times improvement in throughput, 4 times faster time to first token (TTFT), and 2.2 times faster inter-token latency (ITL) compared to the best open-source alternatives.[3]
NVIDIA NIM also integrates seamlessly with Amazon Web Services (AWS) solutions like Amazon SageMaker, Amazon Elastic Kubernetes Service (Amazon EKS), and AWS HealthOmics allowing organizations to develop with familiar toolsets.
When hosting inference solutions on Amazon EKS via NVIDIA NIM, developers can maintain security and control of their generative AI applications by using enterprise-grade software with dedicated feature branches, security patching, rigorous validation processes, and enterprise support that includes access to NVIDIA AI experts. NIM is easy to install, provides full control of underlying model data, and delivers predictable throughput and latency performance.
Learn more about NVIDIA NIM
Right now, businesses in virtually every sector are preparing for a generative AI future. Take the next step and discover how you can deploy and scale generative AI models faster, securely, and more cost-effectively by using NVIDIA NIM through NVIDIA AI Enterprise listed in the AWS Marketplace.
Learn more about NVIDIA NIM on AWS >
[1] “Breakthrough Innovation: Is your organization equipped for breakthrough innovation,” Accenture, 2023
[2] “How to Calculate Business Value and Cost for Generative AI Use Cases,” Gartner, February 2024
[3] “Optimizing Inference Efficiency for LLMs at Scale with NVIDIA NIM Microservices,” NVIDIA, August 2024